Machine Learning is driving huge gains in efficiency and innovation in fraud detection. Behind those gains are Fraud Data Scientists – women and men that train machines to find fraud faster and more accurately by using vast databases of historic transactions to spot patterns of fraud.
I have always admired Fraud Data Scientist because they are smarter and more capable than I.
They also “Make the Magic Happen” – discovering those hidden fraud patterns in mountains of data that no one else can see. Behind almost every text alert, phone call, or notification you receive from a bank about potential fraud on your account is driven by models and scores built by these data scientists.
I Don’t Have Any Good Modeling Techniques For You
To be clear, I have no wise advice on how to use modeling techniques to improve your models. I don’t have a set of code or processes that can boost your model’s fraud detection beyond what you can achieve yourself. I don’t have a magic formula for you.
I am just a business expert. I have never built a model in my life. I do not have a Ph.D. I cannot code. And I am admittedly horrible at math.
So why would you ever listen to me? Because I think I can offer you something different. I use fraud models on a daily basis. I depend on them for my success. I am not a modeler but I am the biggest believer of machine learning out there.
I believe in them so much, I have even helped start up 2 Fraud Analytics Companies. I have seen models fail. And I have seen models exceed expectations wildly. And all the while, I learned new things.
You see, I can’t write a line of code but I do have a secret. The success of your model does not depend on your modeling skills alone. It can depend on other things that you may not have ever considered.
Let me tell you how I think you can boost your model performance now. Here are 7 Ways to Turbo Boost Your Model Performance.
#1 – Be A Fraud Expert First, A Data Scientist Second.
You cannot build a great fraud model if you don’t understand fraud. If you don’t understand how fraud works first, you will struggle when you start to analyze the data.
Don’t get me wrong. You might be able to build a good model that gets a reasonable lift. But I don’t think you can build a GREAT model.
Building a fraud model without understanding the fraud business is what we like to call a “kitchen sink model”. Throw every piece of data into the kitchen sink and just see what the model says. That just doesn’t work.
Become a fraud business expert first before you ever start working on your model. Sit down with fraud investigators and have them walk you through cases. Sit down with fraud analyst and review what they are finding. Interview fraud managers and discover their pain points in fraud. Spend 1-week diving into the intricacies of fraud before you ever open up the database.
Trust me – you will probably build the best fraud model of your life. You will avoid potential target leakers better. You will get ideas on how to better segment your model(s). You will understand how to design your model inputs and outputs so that the model can solve the pain points of the fraud team.
#2 – Put Hidden Fraud Examples Into Your Target Definitions
Any fraud model that exclusively uses fraud tags on detected fraud only is going to be flawed. If you want your models to perform you have to include undetected fraud into your models as well.
If you don’t do this, your models will fall into a self-fulfilling prophecy loop. What that means is that your model will really only predict what fraud analysts have thought was fraud in the past and not what it really was.
What I recommend to avoid this is to bake in alternative fraud tags such as “First Pay Default” or “Early Pay Default” so that you can find fraud that continues to make its way through the system undetected. Experts believe about 70% of early payment defaults or excessively over limit accounts are fraud.
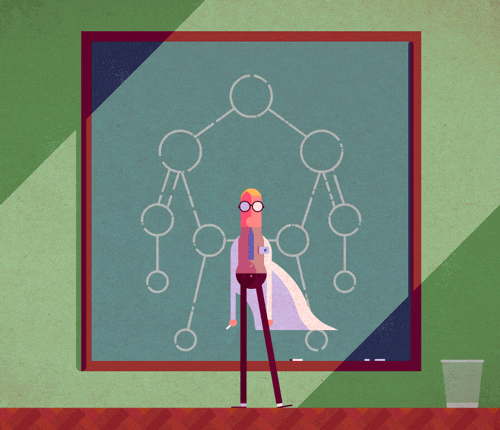
#3 – Build Killer Modeling Presentations.
If you want your model to be a success you have to learn how to communicate the benefit of your model to fraud business experts.
That means PowerPoint or KeyNote should be in your modeling toolkit right along with R, Python and all of the other tools and platforms you use.
Most Data Scientists build their careers on becoming experts on communicating with machines. But they may overlook the fact that their ability to communicate with people is just as important.
If you want to boost your model success, learn how to build killer presentations that describe your model – how you built it, how it works, why it works, what the lift of the model is, what reduction in false positive the business will see and what ROI the model delivers to the business.
Tell people why they should care. Evangelize your Model!
- What data insights did you find that might surprise the fraud analyst?
- What are some of the features in your model that are most important?
- How much data did you use in your model?
- What does your lift chart show in terms of detection? How much better is that than what the analyst do today?
- What is the ROI of using your model?
- What recommendations do you have for use of the model in production?
If you do these things, the odds your model will be a success will increase substantially.
#4 – Build Actionable Output Into Your Fraud Models.
Most models fail because they do not translate to practical actions a business can take based on the model score.
Creating a score that has a correlation to fraud is not enough to make it work. You need to guide the business about what to do with the score.
If you give a Fraud Analyst a fraud score without any context it is just a number. It is meaningless.
There are two ways to make scores mean something.
A. Provide Reason Codes or Alerts – Provide alerts or reason codes that explain the features that had the most weight in the score. What made the score high?
B. Provide Recommended Actions – Based on the reason codes, what should the analyst do with that transaction? What are the steps they should take to mitigate the fraud?
Your model can be a black box, but make sure your output is transparent and clear. Make sure you design actionable output into your model.
#5 – Put Some Cheese on The Broccoli.
When you want a kid to eat broccoli, sometimes you have to put some cheese on it. Broccoli is good for you. Cheese is not. But it’s tasty!
Like broccoli is for kids. We all know that models and scores are good for a Fraud Operation – they can increase efficiency, reduce false positives and help banks and lenders scale their business without needing to hire armies of fraud investigators.
As a Fraud Data Scientist, don’t be afraid to put a little “cheese” your model. You can do that by overlaying some rules that the business experts really want in the score. Maybe those rules will not help the performance. But it will help you get your model accepted. So go ahead, put a little cheese on it.
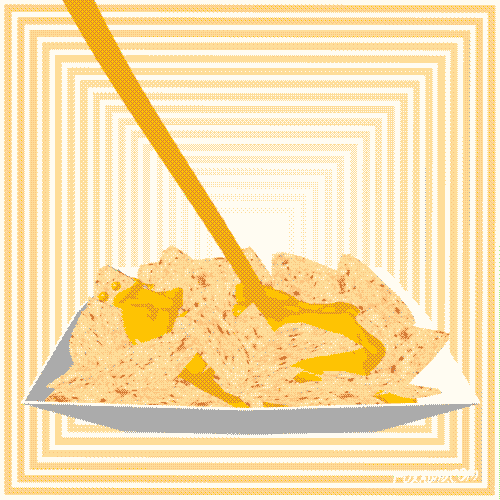
#6 – Guide Your Model Into Operations.
Don’t throw your model over the fence to operations when you are done and hope that it works. There are so many things that can go wrong when you deploy a model:
- The input data could be corrupt or have missing fields that impact the scores.
- The operations group could reject the scores because they don’t understand them.
- The score could require tuning based on production data that you didn’t account for during the model build.
That is why it is important to guide your model into operations. Pilot the score. Turn the score on. Set the highest threshold and work high-risk transactions with the operations. Train them on what the score means so they can make the right interpretations.
When you are convinced that things are running smoothly, then you can move on to your next model.
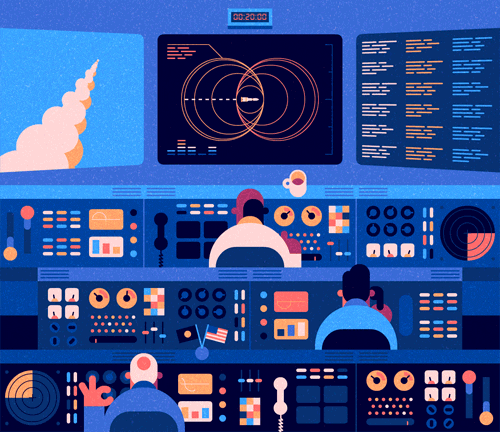
#7 – Improve Your Models Continuously.
The best fraud model was never achieved on the first iteration. In fact, it can take many iterations and retries to get the best performing models.
The best approach I have found is to build several different iterations of the model until you get one you like. Have the scores reviewed by a fraud expert and get their feed back.
Then take that feedback and see if you can improve the results of the model. Never stop improving your models. You can get incrementally better models with time and persistence.
It’s an Art and A Science
Fraud Models are a little bit of art and a lot of science. You have to correctly blend the experience of fraud experts with the logic and statistical background of highly trained fraud data scientist.
It’s the collaboration between business experts and fraud data scientist that really make the models work. If you can achieve that tight collaboration you just might achieve surprising results.
Thank you for reading and let me know if you agree or disagree!