Computing power is doubling every two years. As computers become increasingly powerful, we are finally able to capitalize on the enormous power of artificial intelligence and machine learning.
Self-driving cars, stores without cashiers where you simply walk out with your merchandise and have your card charged automatically, the ability to ask SIRI anything and get an immediate and helpful response; these are all examples of how artificial intelligence has changed our lives in the last 24 months alone.
And it’s just the beginning.
Experts believe in the next 15 years Artificial Intelligence will surpass the human brain in terms of the number of calculations it can do per second. And in 30 years it will surpass all human brains.
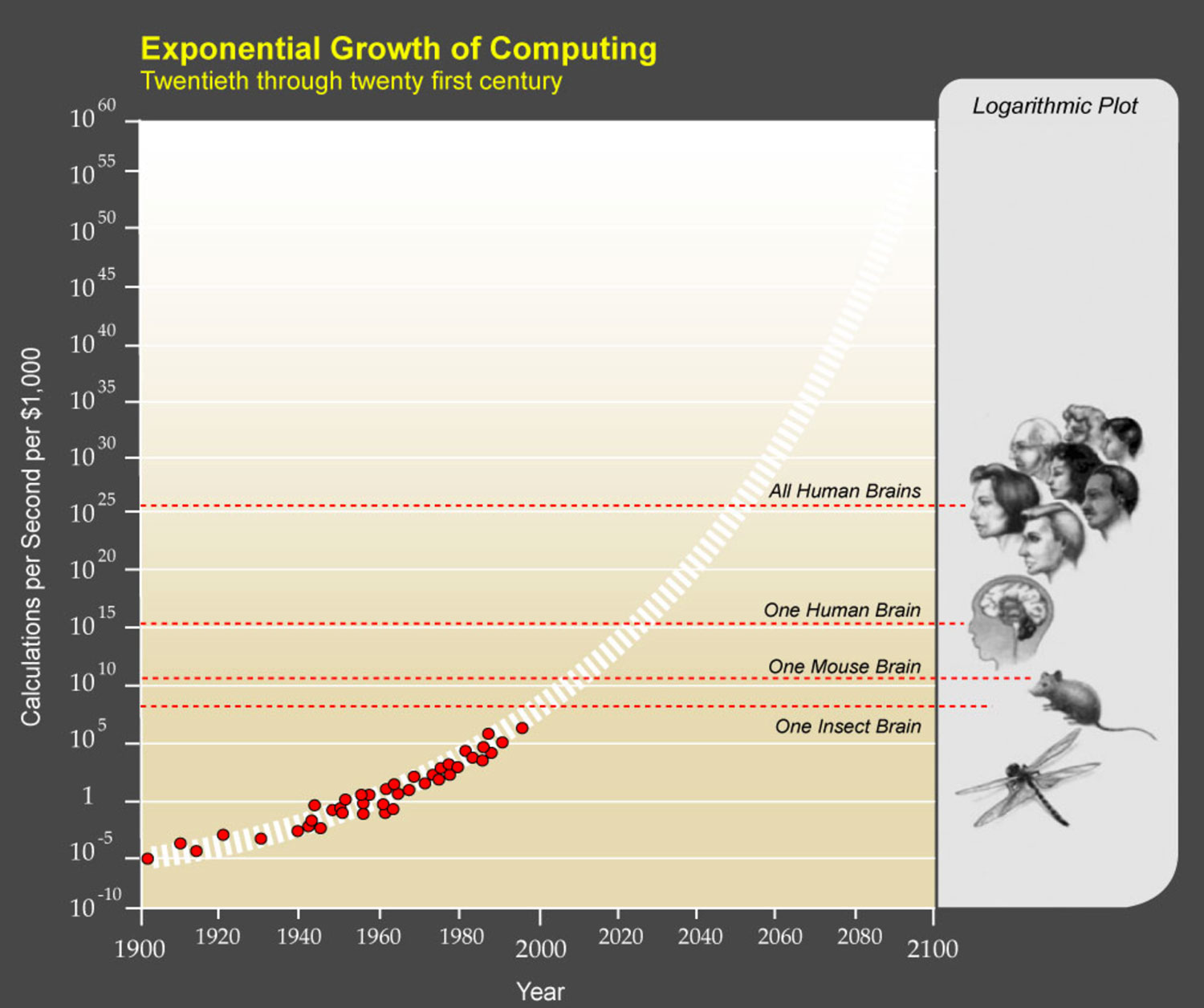
Fraud Machine Learning vs The Human Fraud Analyst
I have been lucky enough to spend my career working for, and eventually starting companies that use fraud machine learning to stop fraud. I often hear people claim that pretty soon these machines will get so good that they can replace people and fully automate fraud detection. I always disagree with them.
You see, I got my start as a fraud analyst. So I know what goes into what they do.
My first job was to review customer’s credit card accounts based on excessive activity. I would work off paper reports that showed customers that had made more than 10 credit card transactions the day before.
I worked tens and thousands of accounts and started to learn fraud pretty well. Eventually, with all that knowledge, I was promoted to Fraud Investigator and then a Fraud Strategy Manager that would write rules based on fraud trends.
I have seen fraud from both the human point of view (as an analyst) as well as from the machine point of view (as a guy that helps build machine learning solutions)
I have learned that you need both approaches to be successful in fraud detection. Machines are only a tool to help the fraud analyst make better decisions. If you try to eliminate the human aspect from fraud detection you are going to lose to the fraudsters who will quickly expose the holes machines cannot see or adapt to.
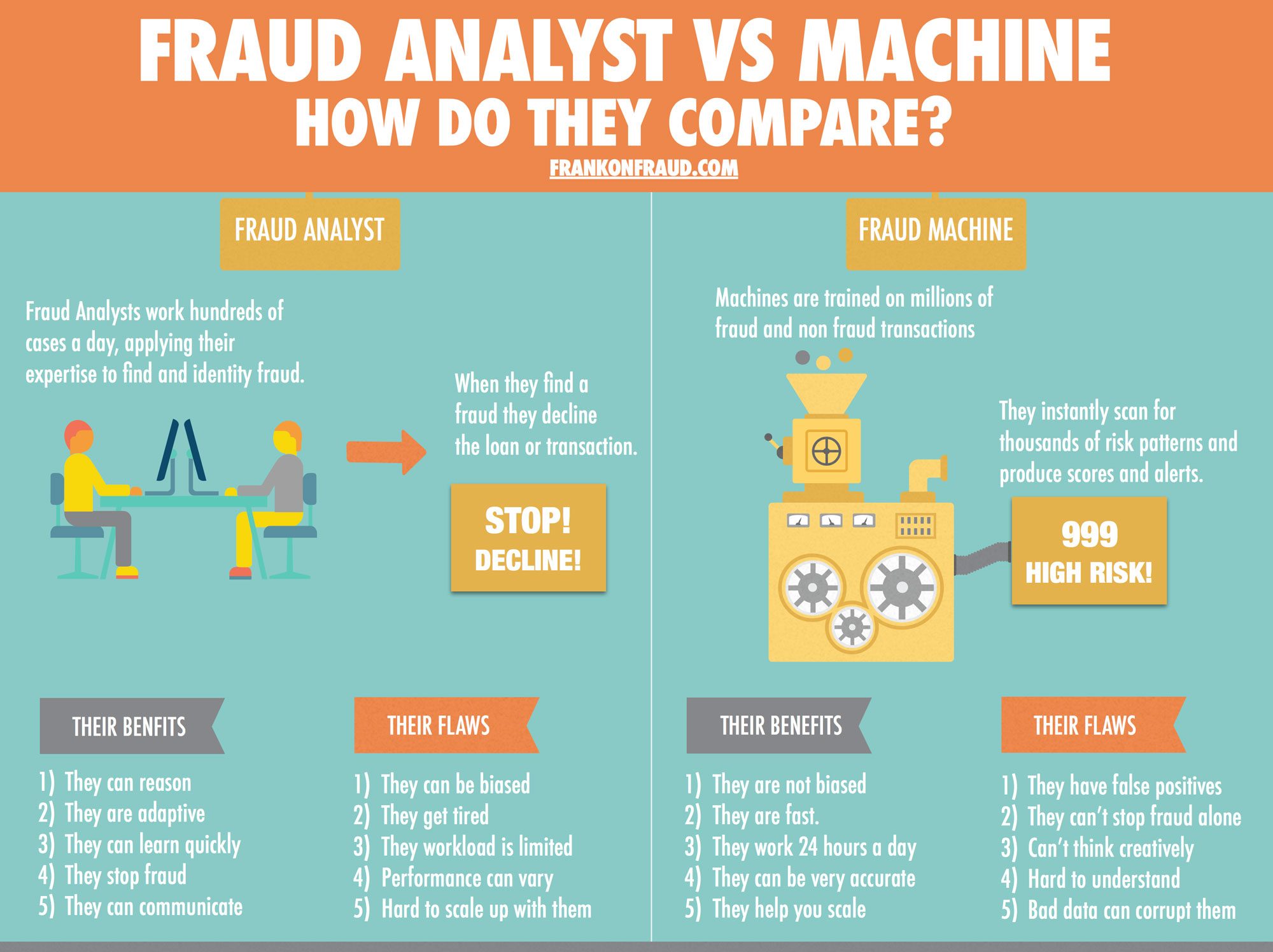
Here are 5 reasons why I believe machines will never eliminate fraud analyst.
#1 – Because Fraud Machines Have False Positives
The weatherman never gets the weather prediction right every time and fraud detection models never predict fraud correctly every time either.
Fraud Models that leverage Artificial Intelligence always have false positives because they make predictions based on probabilities. The probability is never 100% and never 0% since models can never offer certainty. That is why fraud scores are never 0 and never 1000.
As long as there are false positives in models (which will be forever), there will always be a need for fraud analyst to review the output, call customers and make judgment calls.
#2 – Because Fraud Machines Cannot Look Outside Their Box
Fraud machines are fast. They are often very accurate and they are unbiased. But they can only view fraud from the universe of the data they see and were trained on.
Fraud Analysts know how to look beyond what is in front of them to find fraud. They can investigate a document or a transaction that they have never seen before and detect a fraud or forgery by thinking “outside the box”.
This creative ability is what separates a fraud analyst from a fraud machine.
#3 – Because FCRA
In lending and banking, FCRA prohibits the use of many data elements for granting credit. Some of these elements like a zip code cannot be used but they actually offer quite a bit of insight into fraud. Since fraudsters tend to work in networks that are located geographically those views become very important to machine learning models.
Without human fraud analysts to review transactions or loans for fraud, those machine learning models cannot be used because they might unfairly punish people just because of where they live. The fraud analyst reviewing these flagged transactions for fraud enables them to eliminate the false positives and stay compliant.
#4 – Because Fraudsters Exploit Holes in Technology
You need only look at the cat and mouse game of hackers and security to know that fraudsters and scammers will exploit any hole that they can.
Fraud Machine Learning models look for patterns of risk based on history. When those models are used to stop fraud, fraudsters will find and exploit the holes that the model cannot detect.
The fraud analyst acts as the stop gap in these cases and applies hard and fast business rules “Flash Fraud Rules” to help plug the holes of machine learning models.
#5 – Because Machines Don’t Stop Fraud, Fraud Analysts Do
I learned early on that machines don’t stop fraud. People stop fraud. It takes a fraud analyst to interpret the results of the machine learning models and apply the best decision to that score or that alert.
The fraud machine can spit out high-risk alerts and scores all day long but if fraud analyst don’t create the strategies, the processes and the reporting around those high risk alerts the system falls apart.
Machines cannot stop fraud alone, they need their friendly fraud analyst to help them.
You Need Both, Machine and Man
As a former fraud analyst, I can attest to our flaws. We often think that if we could just get our eyes on each and every transaction or each and every loan that we could figure out if it was fraud or not.
But that is just not possible. And it cannot scale. A business cannot grow by hiring armies of fraud analyst to scrutinize every transaction or loan
I have seen the power of models and scores and how they can transform a business. I have seen business save millions of dollars a day with machine learning models.
But I have also seen those models fail when they do not have the right people and processes behind them. The best model is useless if it is not used by the fraud analyst in the right way.
You can’t have machine without man, and man without machine. They are both equally important.
Do you have any others?
Have I missed any other big reasons here? Do you agree with me? What have I missed that I should consider?
Thank you for reading!